As predicted by experts ML will make great progress in 2021. As microcontrollers are becoming more advanced and TinyML is taking it’s hold at the edge.
Embedded machine learning is predicted to achieve the most commonly taken subject in the next year’s as 2021 is near the clock and due to covid-19 situation going on.
Industry watchers predict that despite selicon shortages. On internet of Things devices several new capabilities of embedded machine learning will appear.
New capabilities mean putting an end to the cord between many IoT dives and the cloud, instead the processes will stars running at an edge.
The boost in chip processing capabilities,which will continue to increase next year. Moore’s Law dictates—means sidestepping cloud-based latency among other benefits.
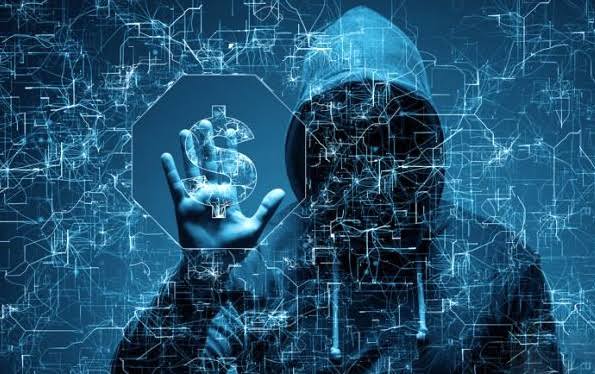
Experts argue that moving processing to the edge – or “going to local execution,” as Hiroshu Doyu, an embedded AI researcher at Ericsson, puts it. Dilevering five distinct advantages in 2021:
- Network bandwidth.
- Network coverage.
- Latency.
- Power consumption.
- Privacy.
Doyu said: privacy will be less “porous” (secure). fewer opportunities for data to be stolen while in transit to the cloud or on the return trip.
“Once the AI is more powerful, that kind of device can be installed without a power line,” Doyu said.
Doyu said : “More powerful IoT AI chips will be shipped and more domain-specific IoT AI chips will be shipped. Both would enable smarter intelligence on IoT sensors,”
Interprice maturation will be forced by proliferation of smarter chips.
“The adoption of way more intelligence at the device level bypasses a lot of the issues, especially with bandwidth and latency,” said Lucy Lee, a senior associate at Volition Capital who tracks embedded AI/ML.
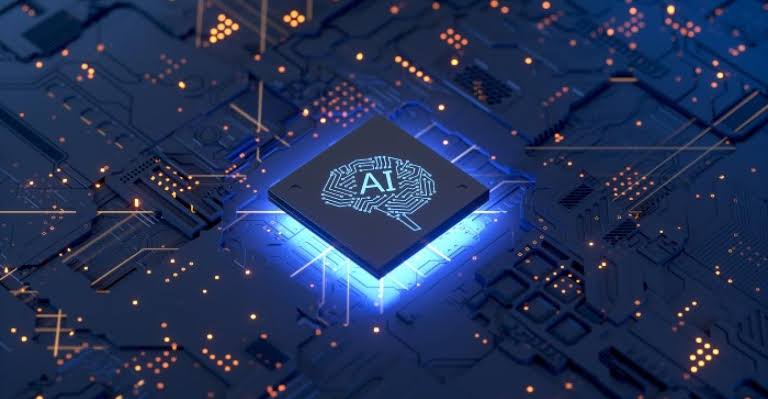
Lucy also predicts that many more autonomous chips will be made in the next year.
But she also stressed that much of it relies on enterprises cleaning up existing systems.
Enterprise IT execs are “completely inundated with the tech stuff we have already embedded. The problem is connecting to it and ingesting it,” Lee said,
It is not feasible to run full scale deep learning models that often have billions of mathematical operations on smaller devices with limited memory and amount.
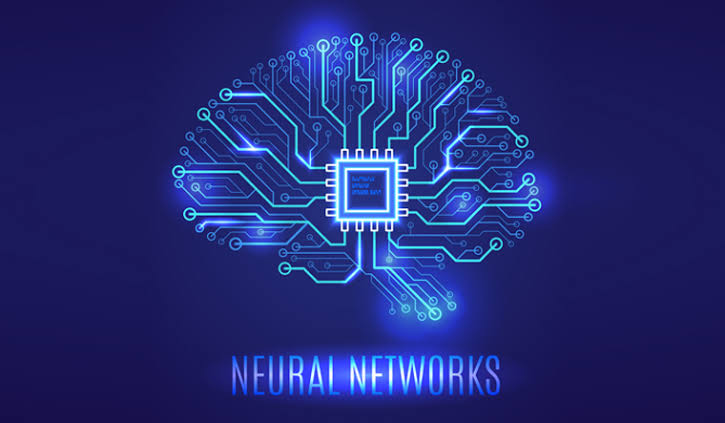
Covid-19 accelerating ML and AI.
COVID-19 Impact: Artificial Intelligence (AI) Chips Market Will Accelerate at a CAGR of Over 42% Through 2020-2024 | Increasing Adoption of AI Chips in Data Centers to Boost Growth.
Chatbots are saving the auto insurance industry during the coronavirus pandemic. Powered by machine learning (ML), digital insurance platforms research applicants’ driving records, analyze data, apply risk metrics to coverage and pricing, and issue policies without face-to-face interaction.
Covid-19 has accelerated the development of machine learning applications that solve today’s problems, according to Professor Daniela Russ.
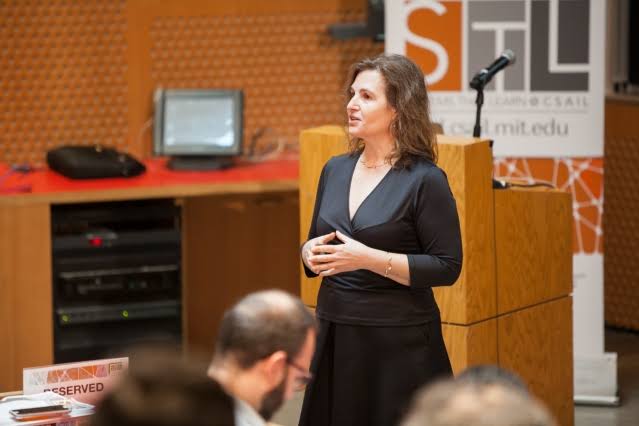
Daniela Russ is the faculty director for MIT’S Computer Science Artificial Intelligence Lab (CSAIL) research alliance. As shared by EETASIA CSAIL launched to develop applications for the latest ML technologies, research challenges limiting ML, and provide professional development for the digital workforce.
CSAIL’s “no idea is too crazy” approach has already yielded numerous breakthroughs in computing technology.
CSAIL currently oversees more than 60 research groups working on hundreds of projects.
EE Times asked Arrow how much it invested in becoming one of the founding members of MIT’s CSAIL research alliance. The company did not respond by press time.
Global spending on artificial intelligence (AI) will reach $97.9 billion in 2023, according to research firm IDC, more than two and one half times the $37.5 billion spent in 2019. The compound annual growth rate (CAGR) for the 2018-2023 forecast period will be 28.4%.
“The pandemic has certainly slowed down the silicon supply chain, as the bulk of global production is based in the APAC region, specifically China and Taiwan, which have been hit particularly hard by the pandemic,” Elman said.
Better Supporting Tools for ML.
In second place for top ML predictions in 2021 sits more comprehensive tool support for ML practitioners.
Today’s ML practitioners demand model interpretability.
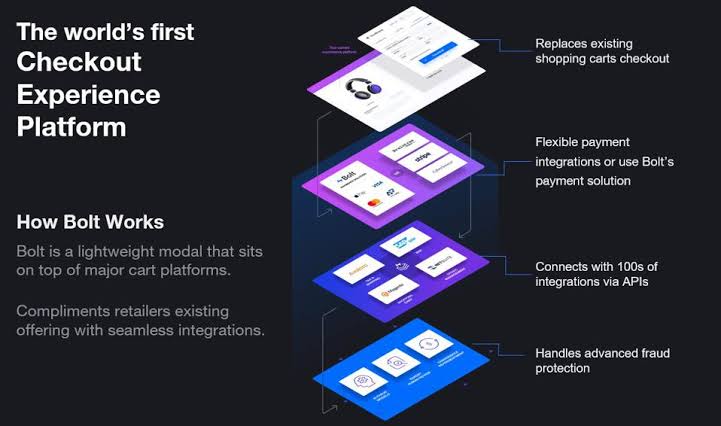
The use of model cards has become a powerful tool for model development, and we expect them to be even more common place in 2021. Essentially these cards, which are more like design documents in practice, formally describe all aspects of a model. Their content can include:
- Detailed Overview: summarizes the model’s purpose
- Specifications: types of layers/neural networks, inputs, and outputs.
- Logistics: authors, date, links to additional documentation, how to cite the model, license.
- Intended Use: applicable use(s), domain constraints, etc.
- Limitations and Considerations: speed/accuracy constraints, ethical and privacy issues, potential for bias, etc.
- Training: data sources, test environments and equipment, etc.
- Target and Actual performance metrics: metrics such as expected versus actual accuracy.
Another key tool is visualization. The ability to visualize a model during design, training, and even in an audit is invaluable in and of itself. This is where PerceptiLabs shines as it offers both a GUI and visual API for TensorFlow.